Distributed Gossip training for Fashion MNIST classification
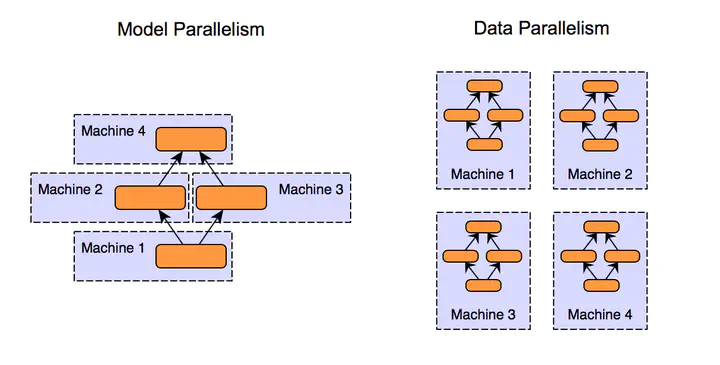
This Project is a Pytorch Implementation of a paper entitled “Gossip training for deep learning” by Michael Blot et al which proposed a distributed method for training deep learning networks.
I’ve used the Gossip training method for a multilabel classification task
Dataset
For implementing gossip training I chose fashion MNIST for training and evaluation of networks. Images of this dataset are gray-level images in 10 classes as described below:
0: T-shirt/top, 1: Trouser, 2: Pullover, 3: Dress, 4: Coat, 5: Sandal, 6: Shirt, 7: Sneaker, 8: Bag, 9: Ankle boot
As a preprocessing step, before Training the networks I normalized the images.
Training
I’ve implemented different Configurations of Gossip training to investigate the role of probability parameters, Communication matrix, and the kind of communication graph.
different models are listed below:
- centralized model
- Gossip training
- Gossip training for different values of parameter
p
as the probability of communication - Gossip training for different communication matrices
- Random Matrix
- Double Random Matix
- The matrix variates with time
- Gossip training for Different Communication graphs
- strongly connected
- periodically strongly connected
- Gossip training for different values of parameter
Below you can see loss and accuracy during training for different amounts of parameter p
:
- Loss during training
- Accuracy during training
Results
Results for different values of parameter p
are in the below table
Parameter p |
Accuracy% |
---|---|
1 | 87,14 |
0.5 | 87,29 |
0.2 | 87,25 |
0.1 | 86,45 |
Results for different Communication matrices are as below
communication matrix | Accuracy% |
---|---|
Random | 90,98 |
Double Random | 90,56 |
Varying with time | 86,94 |
Results for different Communication graphs are as below
communication graph | Accuracy% |
---|---|
Periodically strongly connected | 89,18 |
Strongly connected | 89,81 |